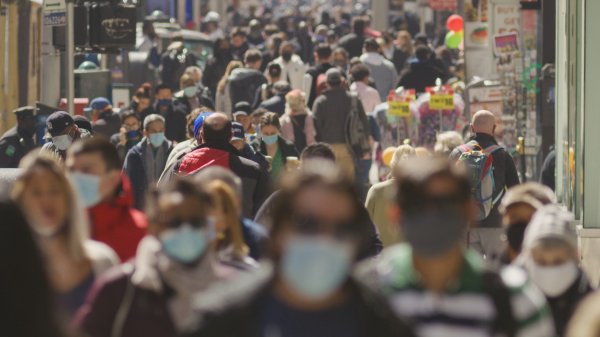
Exposure to air pollution can directly affect human health and increase healthcare use. The World Health Organization (WHO) estimates that 4.2 million deaths annually can be attributed to outdoor air pollution. Urban air pollution assessments have typically relied on a small number of distributed regulatory monitoring stations or land use regression (LUR) models to assess population-scale exposure to air pollution. Most studies showed that the burden of air pollution is disproportionally shared with populations at a higher risk being nonwhite people with pre-existing cardiovascular and respiratory diseases, and people of low socioeconomic status. However, both the sparse spatial distribution and the time lag in these regulatory stations limit the capability of agencies to provide an early warning of a pollution exposure assessment. Recently, communities, researchers, and government agencies have deployed low-cost particulate matter (PM) sensors to provide an alternative approach that increases the spatial and temporal resolutions of the air quality data. The limitations of existing LUR tools are three-fold: 1) the integration of low-cost sensor measurement in such software is not available, 2) the computational efficiencies of such software are notably low, and 3) the model-building process is not interactive and interpretable.
The proposed project aims to develop a modeling framework for air pollution exposure in the top-20 worst air quality metropolitan areas of the US during 2018-2021 by 1) integrating low-cost sensor measurements into the LUR model, 2) creating a robust and interactive machine learning based Pipeline for LUR modeling, and 3) accelerating the LUR model development and model execution.