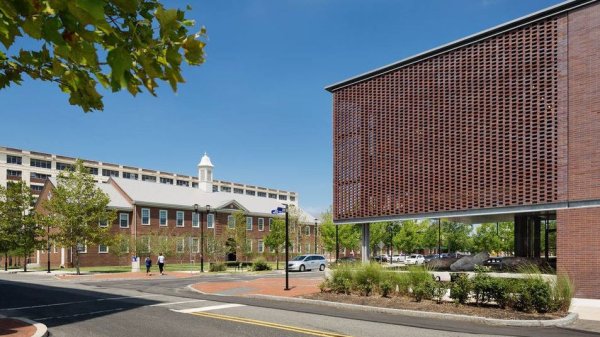
The proposed research is a new collaboration between a junior and senior faculty at Penn State Great Valley and research faculty at Penn State at The Navy Yard, and aims at leveraging the available big data on Penn State buildings at The Philadelphia Navy Yard to: (a) create a comprehensive, open-source database of validated data as the foundation for subsequent analysis in this project and to facilitate research on energy efficient buildings for other researchers and practitioners; (b) develop novel statistical and data visualization tools to analyze historical data, monitor real-time performance, and facilitate scheduling and fault detection; and, (c) develop agent-based simulation models to predict building occupancy and energy consumption, generate expected demand-response scenarios, and enable effective load planning.
The investigators will be working directly with OSIsoft (data infrastructure software provider) and Lutron (lighting control technology provider). Both companies have expressed their support of this project. Future phases involve expanding the agent-based simulation framework to model a smart grid system by using the data on all buildings in The Navy Yard to be provided by the Network Operating Center (legal agreements are already in place). Future extensions also involve development of educational modules for students and professional workforce with the goal to train data analysts that can understand and work effectively with building energy data. Therefore, the proposed project and its future extensions have potentially significant implications for practice, research, and education.
Resulting Publications
- Pallikere, A. (Student Author), Qiu, G., Delgoshaei, P., & Negahban, A., Incorporating occupancy data in scheduling building equipment: A simulation optimization framework. Energy and Buildings, Vol 209, p. 109655. https://doi.org/10.1016/j.enbuild.2019.109655
- Gupta, A. (Student Author), Badr, Y., Negahban, A., & Qiu, G., Energy-Efficient Heating Control for Smart Buildings via Deep Reinforcement Learning. Journal of Building Engineering, Vol 34, p. 101739. https://doi.org/10.1016/j.jobe.2020.101739
- Gupta, A. (MS in Data Analytics, Penn State Great Valley), "Deep Reinforcement Learning Based Energy-Efficient Heating Controller for Smart Buildings." (Thesis already submitted to Penn State Libraries and approved).
- Negahban, A., Pallikere, A., Qiu, G., Delgoshaei, P. (2019). A Simulation Optimization Tool for Incorporating Occupancy Data in Scheduling Building Equipment. Mendeley Data (v1). DOI: doi:10.17632/63pc653jgg.1.
Resulting Presentations
- Negahban, A. (Panelist), April 2018. "Data Analytics and Agent-Based Simulation for Energy Efficient Buildings," PI World Meeting hosted by OSIsoft, San Francisco, CA, Invited Talk, International industry conference.
- Gupta, A. (Student Author and Presenter), Badr, Y., Qiu, G., & Negahban, A., August 19, 2019. "Heating Control in Smart Buildings Enabled by Reinforcement Learning," Artificial Intelligence (AI) for Engineering Summer School, Autodesk, Inc., Toronto, Canada.
- Bhushan, N. (Student Author), Pallikere, A. (Student Author), Megahed, M. (Student Author), Negahban, A., Qiu, G., Wagner, W., & Stutman, M., May 30, 2018 - May 31, 2018. "Energy Efficient Buildings: Using Data Analytics to Incorporate Occupancy in Scheduling and Load Profiling," Energy Days, Penn State, University Park.
Researchers
W. Scott Wagner
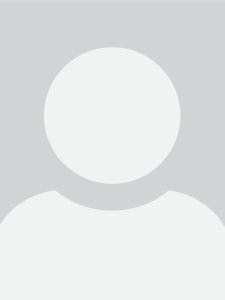