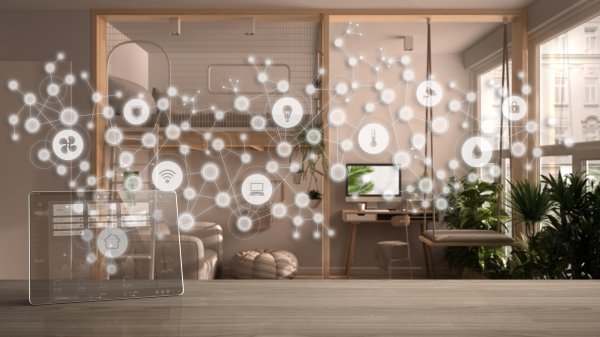
As Americans spend majority of their times (>20 hours daily) indoors, indoor environments play critical roles in human exposure to air pollutants and health implications. The first step to creating a healthy and comfortable indoor environment is to monitor critical indoor air pollutants. Traditionally, indoor air quality sensors have not been integrated into building monitoring systems due to high cost and lack of performance. With recent advances in sensing technology, several low-cost sensors become available for indoor air quality (IAQ) monitoring.
The objectives of this project are to 1) develop an integrated low-cost sensor package that monitors six critical indoor environmental parameters (i.e., CO2, airborne particles, temperature, humidity, light intensity, and acoustics) and can share wirelessly the monitoring data with the cloud system for building controls; 2) conduct “in-situ” sensor performance tests in full-scale occupied buildings; and 3) establish a framework for machine learning based low-cost sensor data analytics that predicts dynamic IAQ profiles depending on occupancy, outdoor conditions, and building operating conditions.
Our interdisciplinary team, led by faculty members from Architectural Engineering and College of Information Sciences and Technology will establish research and teaching framework of IAQ sensor applications and machine learning analytics that can help adjust building operating mode to control IAQ based on time- and space-resolved air quality sensing data. The study approach will provide a solid baseline to characterize indoor air pollution and develop pollutant source reduction measures using low-cost sensing technology in a wide range of building operation and occupancy conditions.