Artificial intelligence (AI) is becoming an integral part of daily life, powering everything from digital assistants to online shopping. But behind this innovation lies a growing environmental footprint. In 2023, data centers consumed 4% of U.S. electricity—a number that could triple by 2028. AI’s rapid expansion also drives higher water usage, emissions, and e-waste, raising urgent sustainability concerns, according to Mahmut Kandemir, a distinguished professor in the Department of Computer Science and Engineering.
Kandemir has spent his career optimizing computer systems for speed and efficiency. Now, he said he sees an unprecedented connection between his research and its environmental impact. To make AI sustainable, he emphasizes the need for proactive solutions—streamlining AI models, developing greener infrastructure, and fostering collaboration across disciplines. In this Q&A, Kandemir discusses how forward-thinking approaches among the tech industry, researchers, and policymakers can ensure that AI continues to drive progress without deepening its environmental footprint.
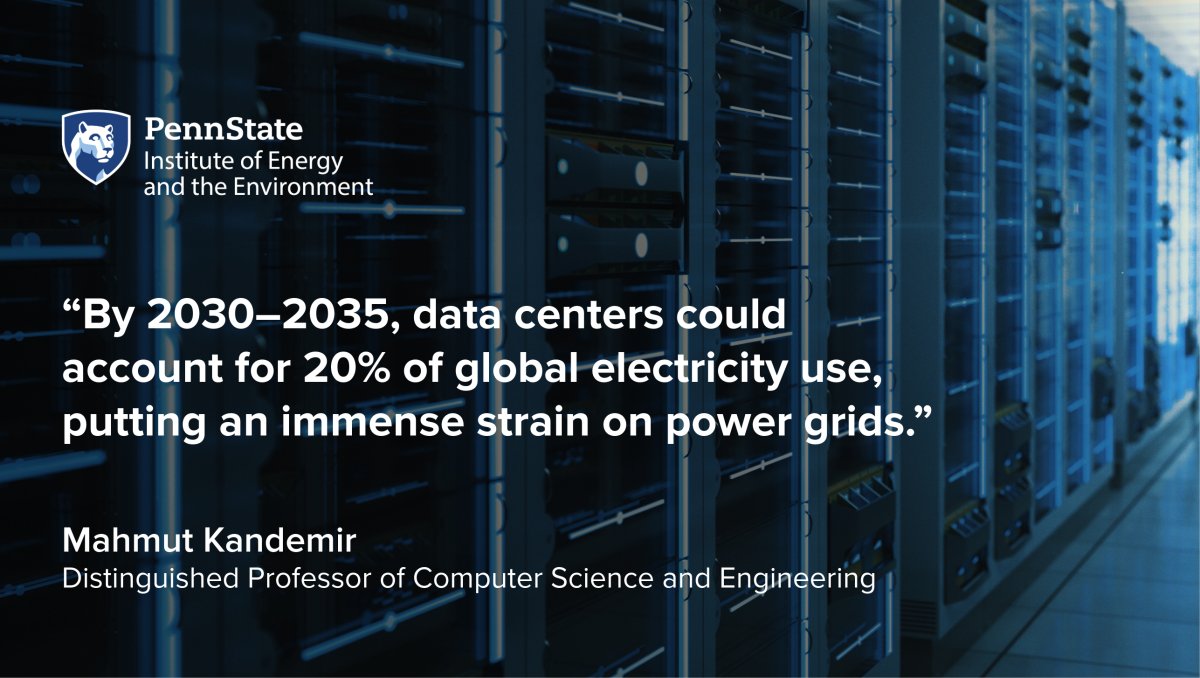
Why is AI’s energy consumption a growing concern?
Initially, energy concerns in computing were consumer-driven, such as improving battery life in mobile devices. Today, the focus is shifting to environmental sustainability, carbon footprint reduction, and making AI models more energy efficient. AI, particularly large language models (LLMs), requires enormous computational resources. Training these models involves thousands of graphics processing units (GPUs) running continuously for months, leading to high electricity consumption. By 2030–2035, data centers could account for 20% of global electricity use, putting an immense strain on power grids.
What makes AI model training so resource-intensive?
AI model training involves training, or adjusting, billions of parameters through repeated computations that require immense processing power. This process demands high-performance computing (HPC) infrastructure, consisting of thousands of GPUs and TPUs (tensor processing units, a specialized chip that improves the speed of machine learning tasks) along with CPUs, all running in parallel. Each training session can take weeks or months, consuming massive amounts of electricity.
Only a handful of organizations, such as Google, Microsoft, and Amazon, can afford to train large-scale models due to the immense costs associated with hardware, electricity, cooling, and maintenance. Smaller institutions with limited GPU/TPU resources would take significantly longer to train models, leading to even higher cumulative energy consumption. Additionally, AI models often require frequent retraining to remain relevant, further increasing energy usage. Infrastructure failures, software inefficiencies, and the growing complexity of AI models add to the strain, making AI training one of the most resource-intensive computing tasks in the modern era.

What are the key environmental consequences of AI development?
The environmental impact of AI extends beyond high electricity usage. AI models consume enormous amounts of fossil-fuel-based electricity, significantly contributing to greenhouse gas emissions. The need for advanced cooling systems in AI data centers also leads to excessive water consumption, which can have serious environmental consequences in regions experiencing water scarcity.
The short lifespan of GPUs and other HPC components results in a growing problem of electronic waste, as obsolete or damaged hardware is frequently discarded. Manufacturing these components requires the extraction of rare earth minerals, a process that depletes natural resources and contributes to environmental degradation.
Additionally, the storage and transfer of massive datasets used in AI training require substantial energy, further increasing AI’s environmental burden. Without proper sustainability measures, the expansion of AI could accelerate ecological harm and worsen climate change.
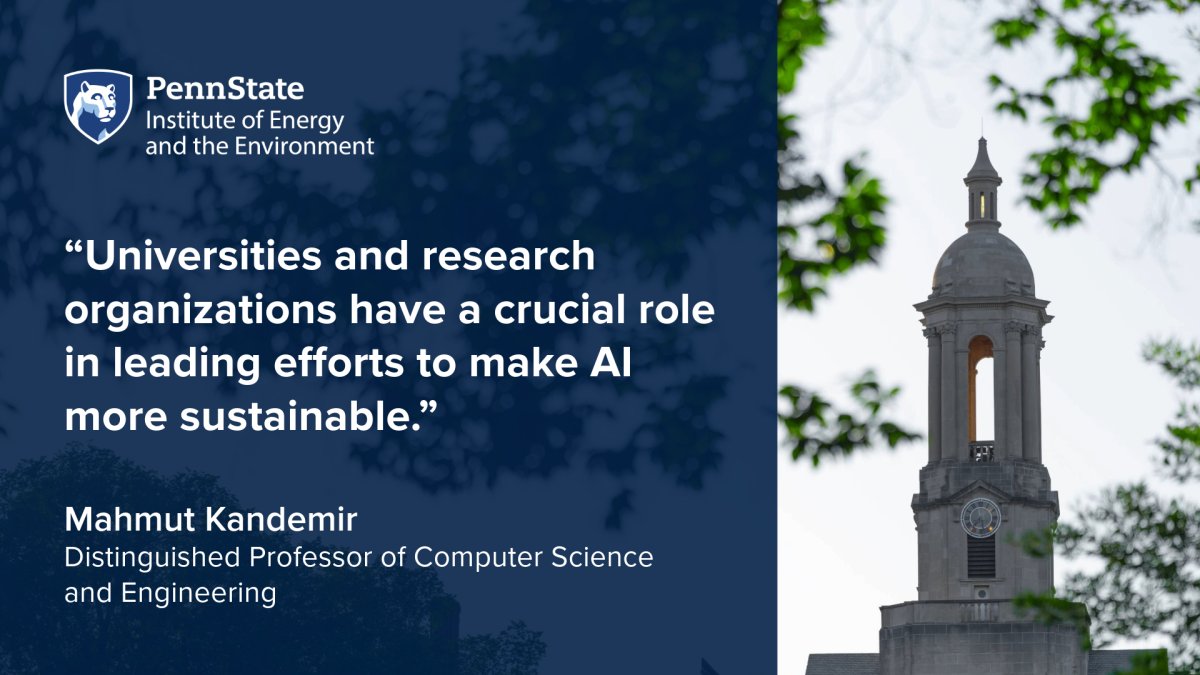
How can AI development become more sustainable?
Several strategies can reduce AI’s environmental footprint while maintaining technological advancements. One approach is to optimize AI models to use fewer resources without significantly compromising performance, making AI more energy efficient. Instead of training large general-purpose models from scratch, researchers can develop domain-specific AI models that are customized for particular fields, such as computational chemistry or healthcare, reducing the computational overhead.
Advancements in hardware can also play a crucial role, as AI-specific accelerators beyond GPUs, such as neuromorphic chips and optical processors, offer the potential for significant energy savings. Additionally, transitioning AI data centers to renewable energy sources like solar and wind can help reduce reliance on fossil fuels, although challenges remain in energy storage and infrastructure adaptation.
Another innovative approach is to distribute AI computations across different time zones, ensuring that computing workloads align with periods of peak renewable energy availability. By implementing these strategies, the AI industry can work toward reducing its environmental impact while continuing to innovate.
What role can research institutions play in AI sustainability?
Universities and research organizations have a crucial role in leading efforts to make AI more sustainable. They can conduct precise carbon footprint assessments of AI workloads to better understand and mitigate the energy impact of AI technologies. Encouraging sustainability through research strategic plans and policy recommendations can push the industry towards greener solutions and influence regulatory decisions. Securing funding from agencies such as the U.S. National Science Foundation (NSF), the Department of Energy (DOE), and the Defense Advanced Research Projects Agency (DARPA), which have placed importance on energy-efficient computing, will be essential in advancing sustainable AI research.
Research institutions can also foster interdisciplinary collaborations among computer scientists, environmental researchers, and policymakers to develop holistic solutions that balance AI progress with environmental responsibility. Additionally, universities can create initiatives such as educational programs, workshops, and public discussions to raise awareness about AI sustainability and encourage the adoption of energy-efficient practices within the AI research community. By taking a proactive role, research institutions can drive meaningful change and help shape a more sustainable future for AI.
Mahmut Kandemir is a distinguished professor of computer science and engineering, and he is an associate director of the Institute for Computational and Data Sciences. He is a member of the Microsystems Design Lab. His research interests are in optimizing compilers, runtime systems, mobile systems, embedded systems, I/O and high-performance storage, nonvolatile processors and memory, and the latest trends in public cloud services.
This content was also published on Penn State News on April 9, 2025.