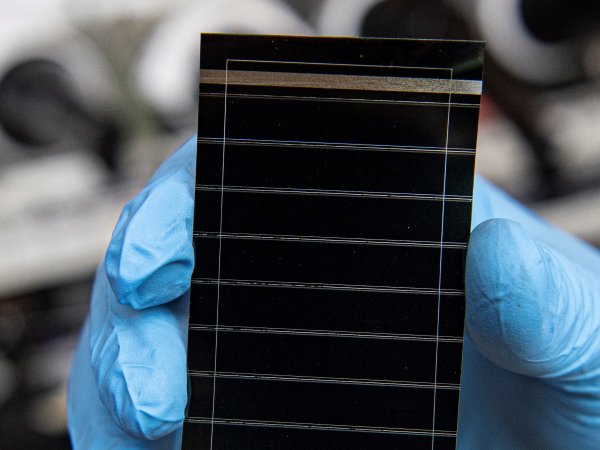
This project delves into the origin of instability of methylammonium lead triiodide (MAPbI3)-based perovskite solar cells (PSCs). Despite the impressive power conversion efficiency (PCE) of PSCs, reaching up to 23% and rivaling mature silicon solar cells, their performance is hindered by instabilities under various environmental and operational conditions. These include temperature variations, moisture, oxygen exposure, and factors intrinsic to device operation, such as bias and light. To address these challenges, the project proposes an innovative approach, combining advanced conductive atomic force microscopy (c-AFM) with state-of-the-art machine learning (ML) techniques to enhance the understanding and control of these instabilities at both macro and nanoscales.
The experimental work centers around the use of c-AFM, an advanced tool for obtaining high-resolution current and topographic images of thin-film materials. These images provide insights into electrical and morphological heterogeneity, surface passivation, chemical modification, and ionic migration, which are critical factors influencing material stability. We plan to utilize c-AFM to investigate the impact of accelerated aging conditions on the electrical conductivity of MAPbI3, exploring the dynamics of optoelectronic deterioration at a scale commensurate with the material's morphology. We will focus on the degradation processes within the grains and at the boundaries, such as phase decomposition and ion migration, crucial for understanding the fundamental causes of MAPbI3 instabilities. State-of-the-art c-AFM equipment at Penn State Behrend will enable the acquisition of high-resolution AFM images complemented by macroscopic current-voltage (I-V) measurements. The image dataset acquired in the proposed project will serve as a valuable resource for future investigations into the correlations between micro and macroscopic phenomena in perovskite thin films and devices.
On machine learning, the project aims to leverage the potential of ML methods in automating the analysis of AFM images. This approach will facilitate the identification of the microstructural evolution of the MAPbI3 thin films under aging conditions in the co-PI Doumon lab, influenced by factors such as moisture, oxygen, and irradiation. The project will apply ML algorithms for unsupervised clustering to establish correlations between the perovskite structure and temporal voltage changes upon light excitation. One of the co-PIs Zhao has expertise in supercomputing and ML models to enhance image analysis. The goal is to comprehensively understand intrinsic (defects, traps) and extrinsic (H2O, O2, bias, temperature, and light) factors affecting PSC performance. This integration of ML with c-AFM analysis will revolutionize the understanding of material and device efficiency recovery during rest periods and extend beyond other perovskite materials diagnosis and characterization.
The novelty of this project lies in its interdisciplinary approach, combining the expertise of Dr. Gong in c- AFM, Dr. Zhao in ML, and Dr. Doumon in solution-processed thin films and devices fabrication and their degradation studies. This collaboration promises significant advancements in perovskite solar cells and offers a model for integrating cutting-edge characterization tools with computational analysis in materials science research. The project's commitment to mentoring undergraduate researchers further underscores its dedication to fostering the next generation of innovators in this rapidly evolving field.